In the post-Moore's Law era , AIFusion ignites a new spark of supercomputing in the field of AI
With the development of science and technology, artificial intelligence (AI) has gradually penetrated into every aspect of our lives. From advanced AI games to the construction of the metaverse, from the realization of autonomous driving to the intelligent operation of big data centers, to the training of large language models like ChatGPT and the application of AI generated content (AIGC), AI application scenarios is expanding at an unprecedented speed and breadth. However, the realization of these applications cannot be achieved without the support of powerful computing power.
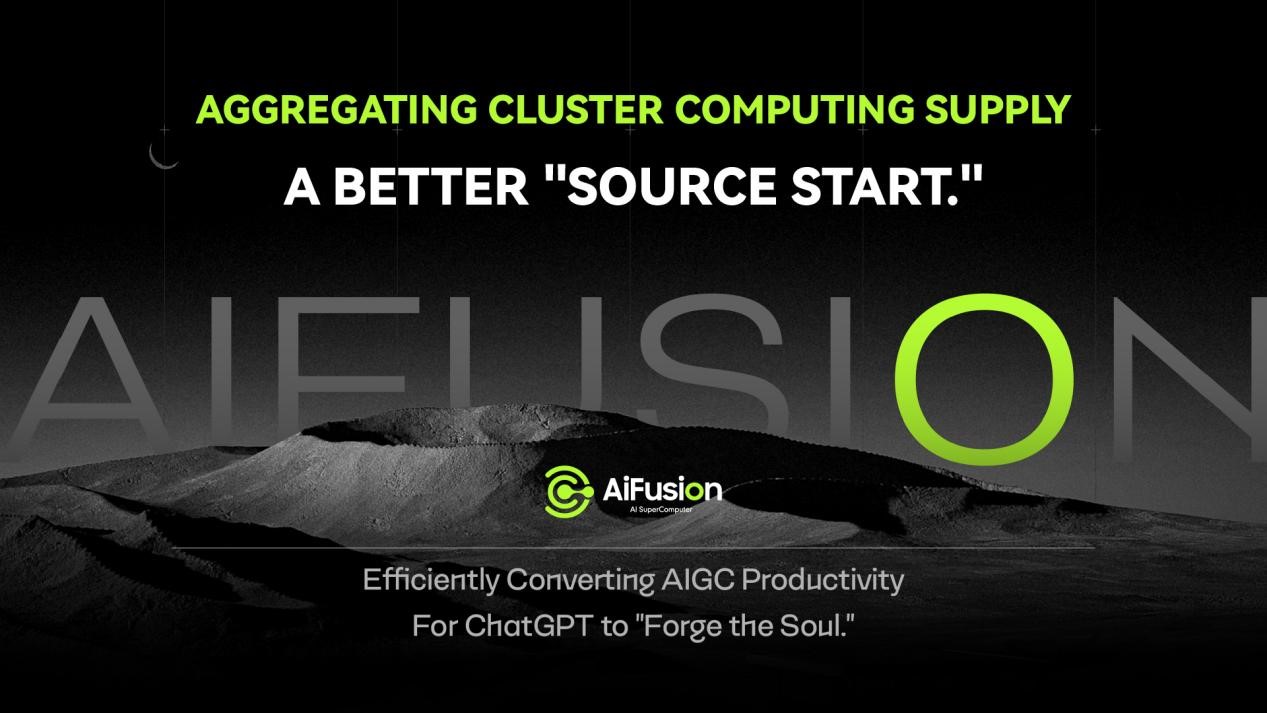
In the PC era, the focus of improving computing power was to increase the main frequency of the CPU, so dual-core and multi-core heterogeneous systems became popular. From the PC era to the era of mobile terminals such as mobile phones and tablets, higher requirements have been put forward for multimedia and graphics rendering, so GPUs have become more and more important. Compared with CPU, GPU's floating point calculation method is more suitable for the calculation of AI algorithm model. In fact, the early hardware foundation of many AI applications is GPU, which uses GPU to run a variety of general-purpose AI algorithms.
In the early Moore's Law era, AI generally adopted the von Neumann architecture that separated computing and storage, which was characterized by low computing power and low energy consumption ratio. At that time , CPU chips represented by Intel , AMD, and ARM were the crown jewels of integrated circuits. With the emergence of GPUs, AI acceleration chips such as NPU and other processors, and the advent of the post-Moore era, computing power has begun to increase significantly, but the energy consumption ratio is still low. For example, the mining needs of blockchain and digital currencies have promoted the replacement of GPUs with single hardware accelerators. Today, whether it is autonomous driving, metaverse or AIGC applications, the cost of using GPUs is already too high. AI manufacturers prefer to design their own ASIC chips and use dedicated computing architecture, which can bring about several times or even dozens of times the energy consumption ratio.
Deep learning in AI requires powerful parallel processing capabilities. Therefore, AI processors are mainly concentrated in GPUs, FPGAs, ASICs, and NPUs, and manufacturers are also developing and upgrading these processors to meet the challenges. Among them, GPU is currently the most important AI processor. In the first half of 2021, in China's AI chip market, GPU accounted for more than 90%, and ASIC, FPGA, NPU, etc. accounted for nearly 10%. It is expected that the share will exceed 20% in 2025.
There are various application scenarios for AI, such as the Metaverse, autonomous driving, and AIGC. Different application requirements require different computing power.
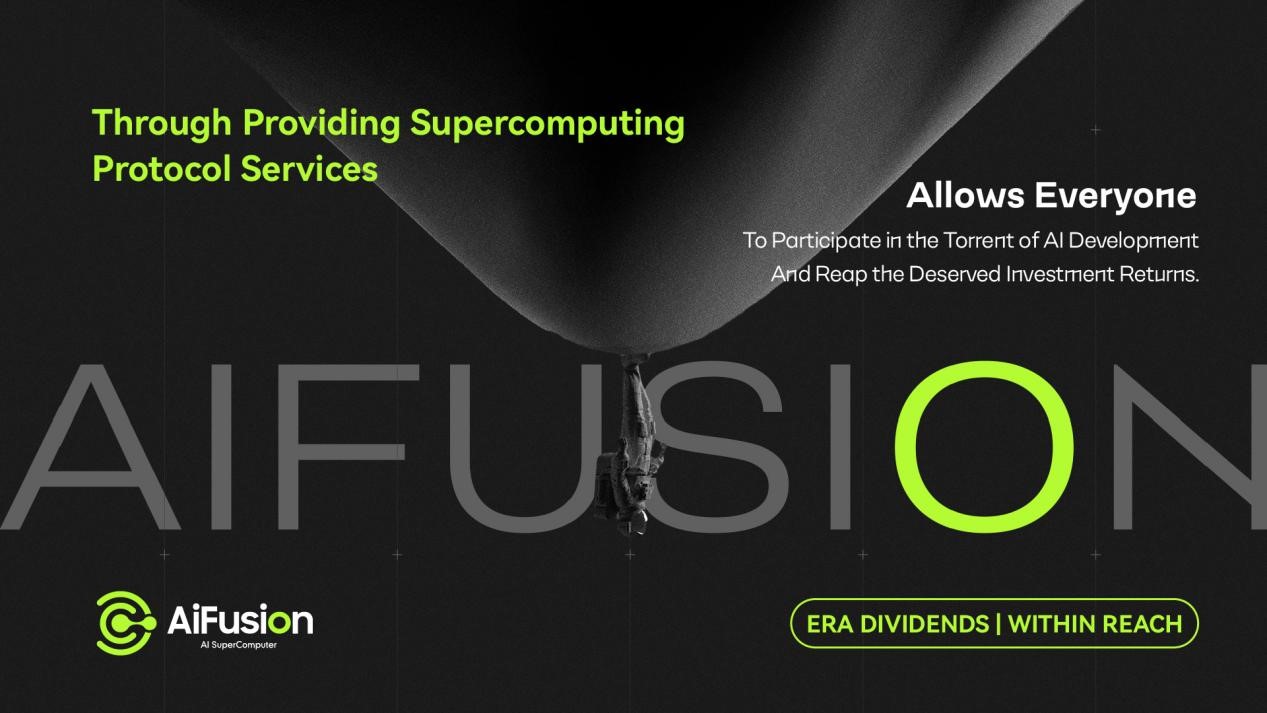
The surge in demand for computing power caused by AIGC
AIGC (AI generated content) is artificial intelligence generated content, also known as generative AI. It refers to the use of artificial intelligence technology to generate content, including text, voice, code, images, videos, robot actions, etc. Currently, the most popular AIGC applications are large language models such as ChatGPT. The training and inference of such models require a large amount of computing resources, especially during the training phase, which places great demand on GPUs and other high-performance computing devices.
The popularity of ChatGPT marks the start of commercialization of AIGC. It promotes the in-depth development of AI based on large-scale neural networks, and the demand for computing power also surges simultaneously. Model training is the core challenge, and the complexity of algorithm models increases exponentially. Taking GPT3 as an example, training on a corpus of 175 billion parameters and 100 billion words requires 1,000 Nvidia A100 GPUs for one month of training. In less than a year, the trillion-parameter language model Switch Transformer came out. Artificial intelligence computing power doubles every two months, and supply levels affect AI innovation and industrial application implementation. AI models have entered the trillion-level era, and deep learning has entered the stage of large models and big data. The demand for computing power exceeds the actual growth rate by 375 times every two years.
There are reports that OpenAI requires a daily operating cost of US$694,444 and approximately 3,617 HGX A100 servers to provide services for ChatGPT.
ChatGPT is based on Transformer technology, and its operation requires large-scale pre-training and a large amount of computing power. This not only drives the demand for AI underlying chips, but also drives the demand for high-end chips. With the popularization of ChatGPT applications in various fields, such as smart speakers, game NPCs, etc., and the increase in frequency of use, the demand for AI servers has also increased dramatically. The global AI server market is expected to reach US$28.8 billion in 2025.
AI servers are mainly in the form of CPU+GPU and support large-scale neural network models. my country's server manufacturers are in a leading position in the world and have broad room for market growth. In the past five years, the domestic computing power industry has grown by more than 30%, ranking second in the world. It has entered the era of more than 1000TOPS computing power, and data centers and supercomputers are typical scenarios. The "Three-Year Action Plan for the Development of New Data Centers" states that the total computing power will exceed 200 EFLOPS by the end of 2023, and will exceed 300 EFLOPS by 2025. The supercomputing center is developing towards Z-level computing power, and E-level computing is the world's top supercomputing goal. Taking the Frontier Supercomputing Center of Oak Ridge Laboratory in the United States as an example, its double-precision floating point computing power reaches 1.1 EOPS.
In addition to the CPU+GPU form, because FPGA has the characteristics of high flexibility and short development cycle, while ASIC has the advantages of small size and low power consumption, they are also used in AI servers. Especially in fields such as machine learning and edge computing, ASIC has received widespread attention. Google's TPU v4 and Baidu's Kunlun 2nd generation AI chip have achieved remarkable results in this direction.
It is worth noting that as the demand for cloud chip processing increases, the pressure on the cloud continues to increase, driving the trend of AI processing moving to the edge. The resulting changes in user needs are also driving changes in the division of labor in AI chips. On the one hand, they want to protect data privacy, and on the other hand, they need to process results in a timely manner. Therefore, the focus of AI processing is accelerating to shift to the edge.
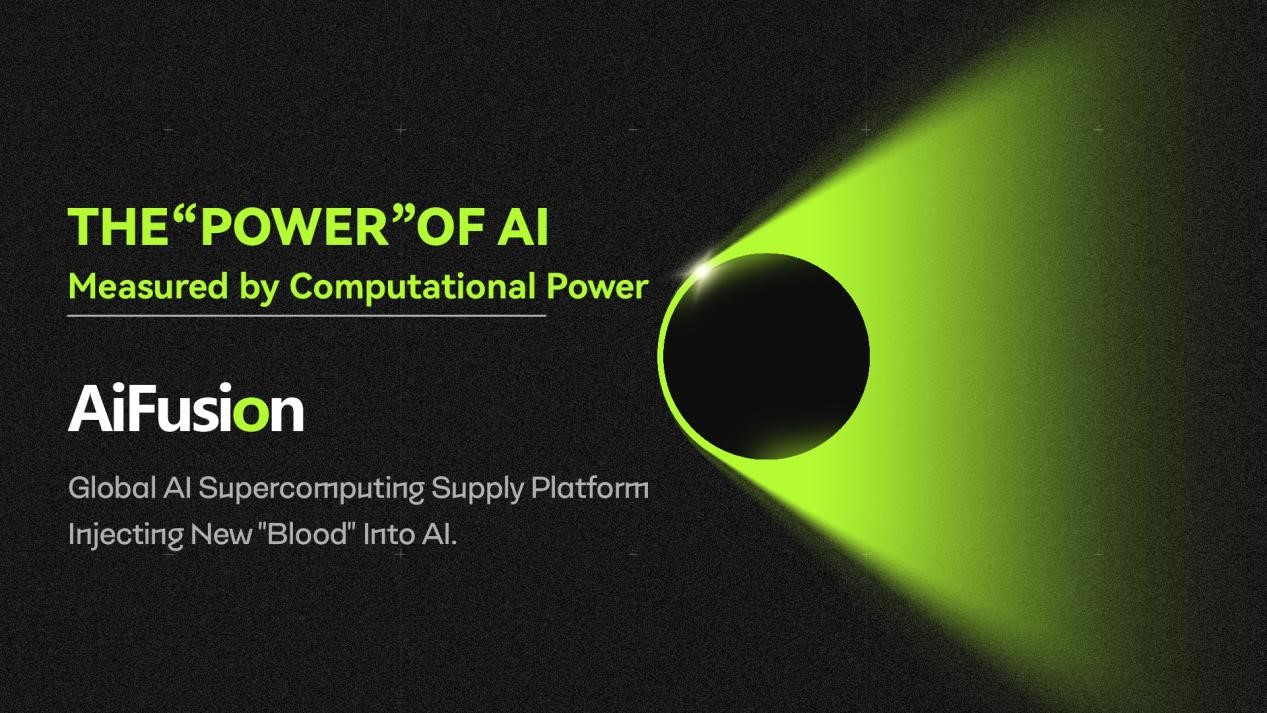
AI computing power growth and challenges in the post-Moore’s Law era
In the past, chip computing power was determined by data interconnection, unit transistor computing power, transistor density and chip area. Therefore, to improve chip computing power, it is mainly achieved by improving the system architecture, using more advanced processes to increase transistor density, using distributed computing and heterogeneous computing, and adopting new chiplet packaging.
However, Moore's Law has become saturated in recent years and cannot sustain such rapid growth. Against this background, the computing power demand for intelligent computing has increased six times in seven years. Take image recognition and natural language processing as examples. Behind their performance improvements is the exponential growth in demand for computing power. An example of this explosive growth in demand is the GPT series of models. From GPT-1 to GPT-4, the computing power has increased thousands of times in just 5 years.
In the post-Moore's Law era, the growth of AI computing power not only relies on the improvement of hardware, as described by Moore's Law, but also relies on the optimization of software and algorithms, and the data transmission rate has also become a computing power bottleneck. The efficiency of algorithms and the effective utilization of computing architecture play a key role in achieving performance improvements.
The computing architecture of AI processors needs to change with the development of the times to adapt to evolving needs. Future AI processors not only need to provide powerful computing capabilities, but also need to consider factors such as power consumption, size, and cost. At the same time, the collaborative optimization of software and hardware will also become the key to improving AI performance.
Currently, the development of AI has penetrated into all areas of our lives, and the demand for computing power is also growing. In the future, we will see more innovations in AI processors, including GPUs, NPUs, and ASICs, to meet this growth in demand and promote the further development of AI technology.
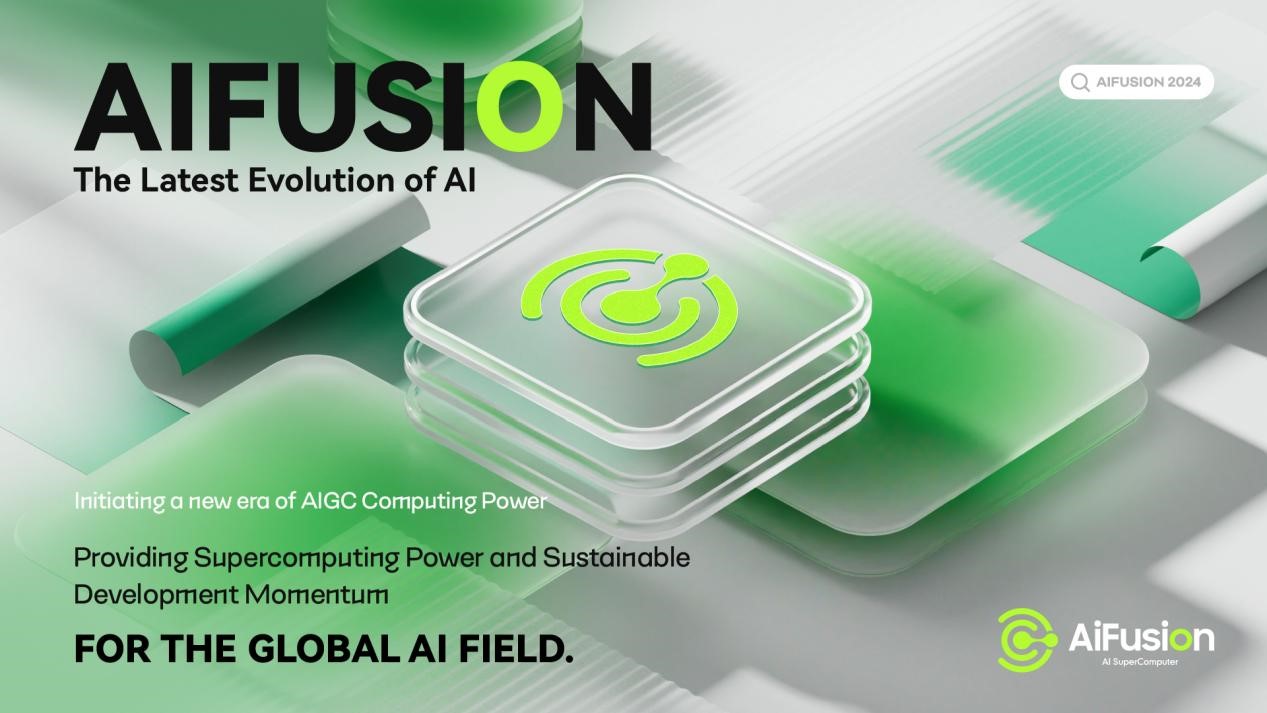
AIFusion: igniting a new supercomputing spark in the field of AI
Driven by the rapid development of technology and the trend of artificial intelligence, AIFusion was born, aiming to provide unprecedented cheap computing power and sustainable development super power for global AIGC entrepreneurs and developers in the AI field. This marks the official beginning of the supercomputing revolution in the AI era, injecting new vitality into the entire artificial intelligence industry.
AIFusion is not only a platform, but also a global experiment, committed to promoting the development of the artificial intelligence industry through its unique distributed computing power cluster network and computing power rental services under a trusted network. The platform provides global investors with convenient participation methods and AI income paths, and provides AIGC entrepreneurs and developers with an innovative and efficient computing power support system.
The AIFusion supercomputing protocol is at the heart of this revolutionary experiment. This agreement allows users to purchase AI supercomputing network mapping servers, follow the mining distribution mechanism of the agreement, and enjoy the computing power income dividends generated by the corresponding servers. This unique incentive mechanism not only allows users to fully enjoy advanced computing power services, but also becomes a powerful participant in the core computing power supply chain of the global AIGC industry.
AI Supercomputer, as a distributed supercomputing network platform launched by AIFusion, is jointly built by AIFusion Labs and Inflection AI, the founder of the foundation. The platform purchases computing power through centralized supercomputing equipment suppliers in 20 countries and regions around the world, providing stable and low-cost computing power rental, technology expansion and analytical data business support for AIGC industrial institutions and AI generation systems. This provides global users with the opportunity to participate in the core computing power supply chain of the AIGC industry, allowing them to obtain considerable returns on investment in the computing power blue ocean market.
The launch of the AIFusion supercomputing protocol means that global investors have a more open, inclusive, and shared opportunity to participate more deeply in the core of the artificial intelligence industry. This is not only a technological change, but also a revolution that connects the world with new sparks of AI supercomputing.
Under the leadership of AIFusion, we have witnessed the supercomputing revolution in the AI era and look forward to this innovative and dynamic platform bringing more surprises and breakthroughs to the field of artificial intelligence. AIFusion helps AI dreams soar and creates a new era of supercomputing!
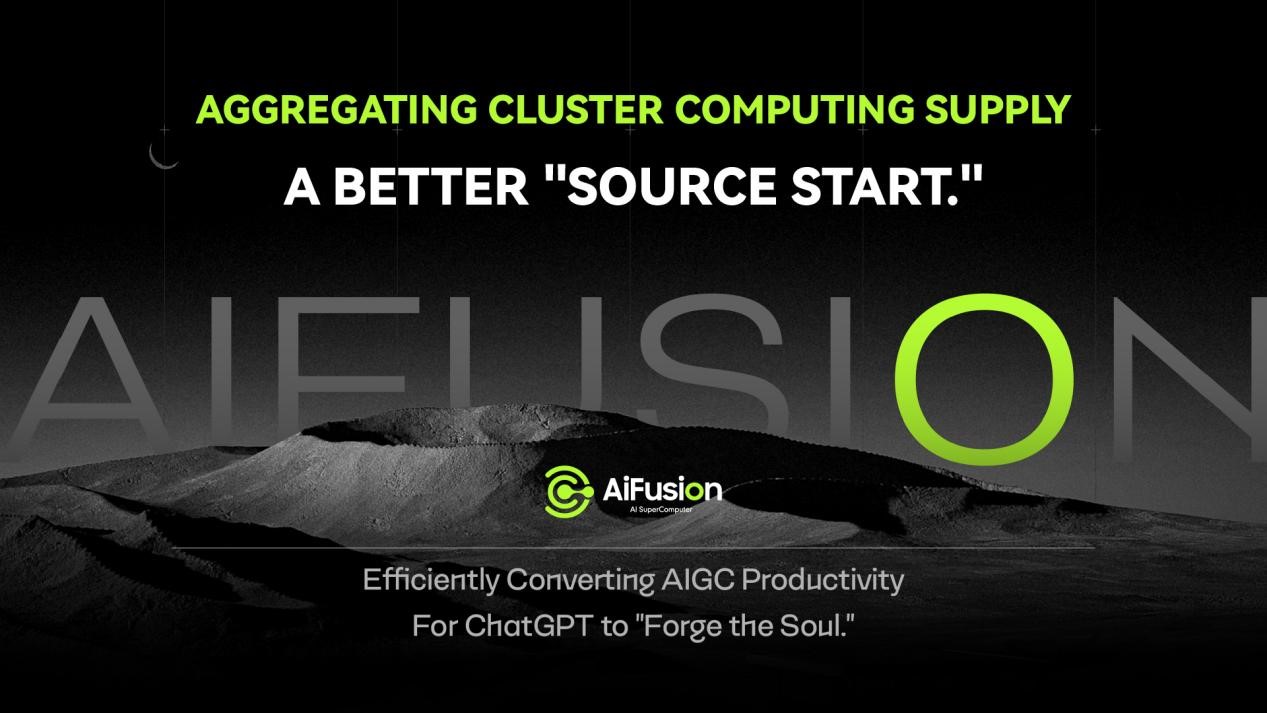
In the PC era, the focus of improving computing power was to increase the main frequency of the CPU, so dual-core and multi-core heterogeneous systems became popular. From the PC era to the era of mobile terminals such as mobile phones and tablets, higher requirements have been put forward for multimedia and graphics rendering, so GPUs have become more and more important. Compared with CPU, GPU's floating point calculation method is more suitable for the calculation of AI algorithm model. In fact, the early hardware foundation of many AI applications is GPU, which uses GPU to run a variety of general-purpose AI algorithms.
In the early Moore's Law era, AI generally adopted the von Neumann architecture that separated computing and storage, which was characterized by low computing power and low energy consumption ratio. At that time , CPU chips represented by Intel , AMD, and ARM were the crown jewels of integrated circuits. With the emergence of GPUs, AI acceleration chips such as NPU and other processors, and the advent of the post-Moore era, computing power has begun to increase significantly, but the energy consumption ratio is still low. For example, the mining needs of blockchain and digital currencies have promoted the replacement of GPUs with single hardware accelerators. Today, whether it is autonomous driving, metaverse or AIGC applications, the cost of using GPUs is already too high. AI manufacturers prefer to design their own ASIC chips and use dedicated computing architecture, which can bring about several times or even dozens of times the energy consumption ratio.
Deep learning in AI requires powerful parallel processing capabilities. Therefore, AI processors are mainly concentrated in GPUs, FPGAs, ASICs, and NPUs, and manufacturers are also developing and upgrading these processors to meet the challenges. Among them, GPU is currently the most important AI processor. In the first half of 2021, in China's AI chip market, GPU accounted for more than 90%, and ASIC, FPGA, NPU, etc. accounted for nearly 10%. It is expected that the share will exceed 20% in 2025.
There are various application scenarios for AI, such as the Metaverse, autonomous driving, and AIGC. Different application requirements require different computing power.
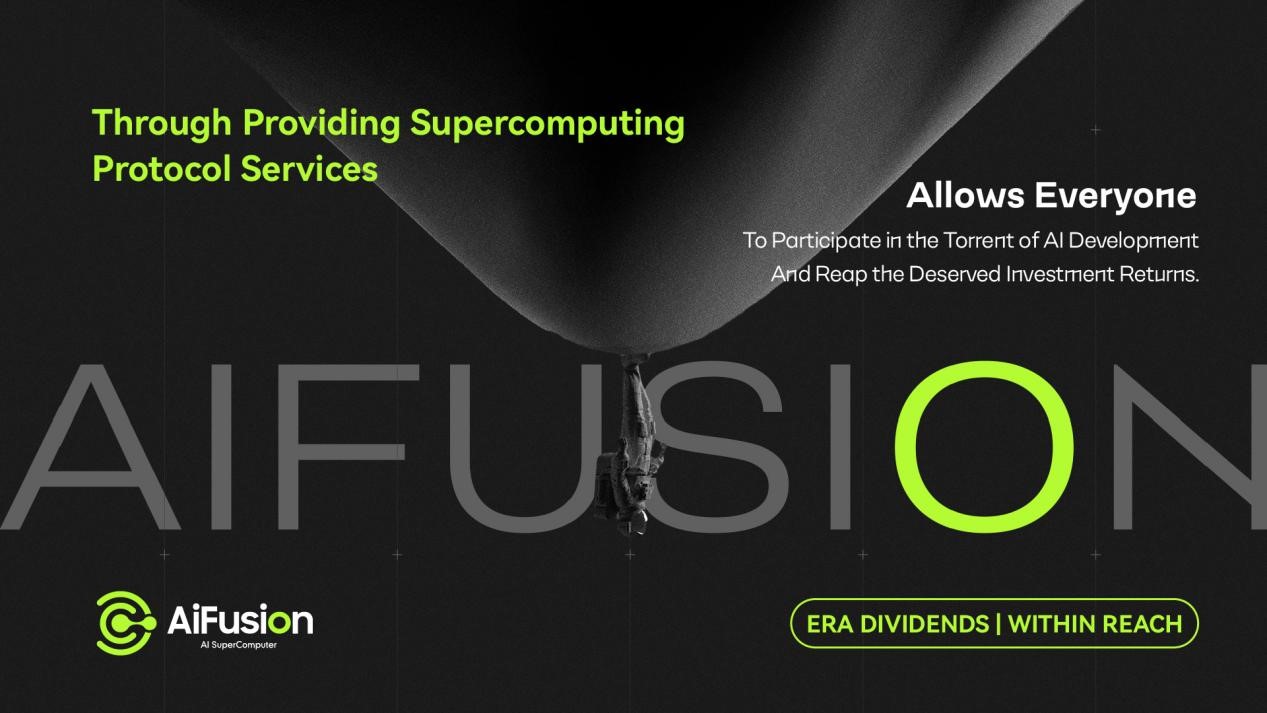
The surge in demand for computing power caused by AIGC
AIGC (AI generated content) is artificial intelligence generated content, also known as generative AI. It refers to the use of artificial intelligence technology to generate content, including text, voice, code, images, videos, robot actions, etc. Currently, the most popular AIGC applications are large language models such as ChatGPT. The training and inference of such models require a large amount of computing resources, especially during the training phase, which places great demand on GPUs and other high-performance computing devices.
The popularity of ChatGPT marks the start of commercialization of AIGC. It promotes the in-depth development of AI based on large-scale neural networks, and the demand for computing power also surges simultaneously. Model training is the core challenge, and the complexity of algorithm models increases exponentially. Taking GPT3 as an example, training on a corpus of 175 billion parameters and 100 billion words requires 1,000 Nvidia A100 GPUs for one month of training. In less than a year, the trillion-parameter language model Switch Transformer came out. Artificial intelligence computing power doubles every two months, and supply levels affect AI innovation and industrial application implementation. AI models have entered the trillion-level era, and deep learning has entered the stage of large models and big data. The demand for computing power exceeds the actual growth rate by 375 times every two years.
There are reports that OpenAI requires a daily operating cost of US$694,444 and approximately 3,617 HGX A100 servers to provide services for ChatGPT.
ChatGPT is based on Transformer technology, and its operation requires large-scale pre-training and a large amount of computing power. This not only drives the demand for AI underlying chips, but also drives the demand for high-end chips. With the popularization of ChatGPT applications in various fields, such as smart speakers, game NPCs, etc., and the increase in frequency of use, the demand for AI servers has also increased dramatically. The global AI server market is expected to reach US$28.8 billion in 2025.
AI servers are mainly in the form of CPU+GPU and support large-scale neural network models. my country's server manufacturers are in a leading position in the world and have broad room for market growth. In the past five years, the domestic computing power industry has grown by more than 30%, ranking second in the world. It has entered the era of more than 1000TOPS computing power, and data centers and supercomputers are typical scenarios. The "Three-Year Action Plan for the Development of New Data Centers" states that the total computing power will exceed 200 EFLOPS by the end of 2023, and will exceed 300 EFLOPS by 2025. The supercomputing center is developing towards Z-level computing power, and E-level computing is the world's top supercomputing goal. Taking the Frontier Supercomputing Center of Oak Ridge Laboratory in the United States as an example, its double-precision floating point computing power reaches 1.1 EOPS.
In addition to the CPU+GPU form, because FPGA has the characteristics of high flexibility and short development cycle, while ASIC has the advantages of small size and low power consumption, they are also used in AI servers. Especially in fields such as machine learning and edge computing, ASIC has received widespread attention. Google's TPU v4 and Baidu's Kunlun 2nd generation AI chip have achieved remarkable results in this direction.
It is worth noting that as the demand for cloud chip processing increases, the pressure on the cloud continues to increase, driving the trend of AI processing moving to the edge. The resulting changes in user needs are also driving changes in the division of labor in AI chips. On the one hand, they want to protect data privacy, and on the other hand, they need to process results in a timely manner. Therefore, the focus of AI processing is accelerating to shift to the edge.
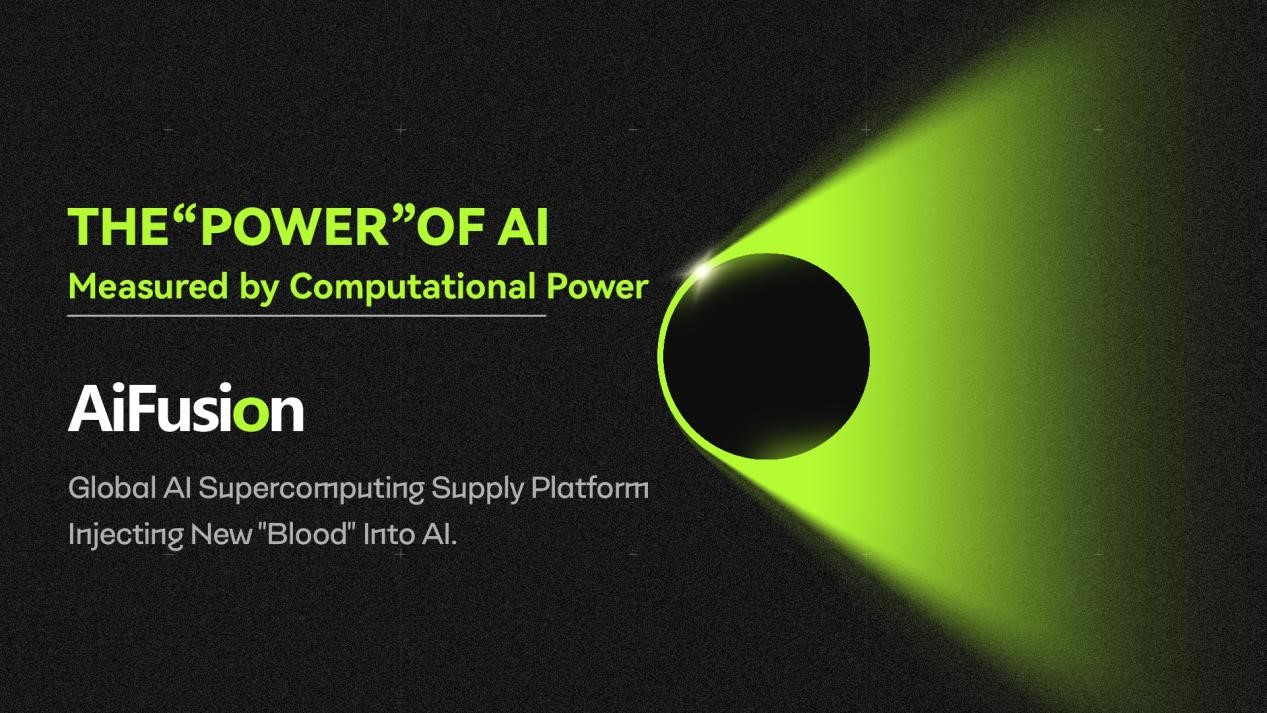
AI computing power growth and challenges in the post-Moore’s Law era
In the past, chip computing power was determined by data interconnection, unit transistor computing power, transistor density and chip area. Therefore, to improve chip computing power, it is mainly achieved by improving the system architecture, using more advanced processes to increase transistor density, using distributed computing and heterogeneous computing, and adopting new chiplet packaging.
However, Moore's Law has become saturated in recent years and cannot sustain such rapid growth. Against this background, the computing power demand for intelligent computing has increased six times in seven years. Take image recognition and natural language processing as examples. Behind their performance improvements is the exponential growth in demand for computing power. An example of this explosive growth in demand is the GPT series of models. From GPT-1 to GPT-4, the computing power has increased thousands of times in just 5 years.
In the post-Moore's Law era, the growth of AI computing power not only relies on the improvement of hardware, as described by Moore's Law, but also relies on the optimization of software and algorithms, and the data transmission rate has also become a computing power bottleneck. The efficiency of algorithms and the effective utilization of computing architecture play a key role in achieving performance improvements.
The computing architecture of AI processors needs to change with the development of the times to adapt to evolving needs. Future AI processors not only need to provide powerful computing capabilities, but also need to consider factors such as power consumption, size, and cost. At the same time, the collaborative optimization of software and hardware will also become the key to improving AI performance.
Currently, the development of AI has penetrated into all areas of our lives, and the demand for computing power is also growing. In the future, we will see more innovations in AI processors, including GPUs, NPUs, and ASICs, to meet this growth in demand and promote the further development of AI technology.
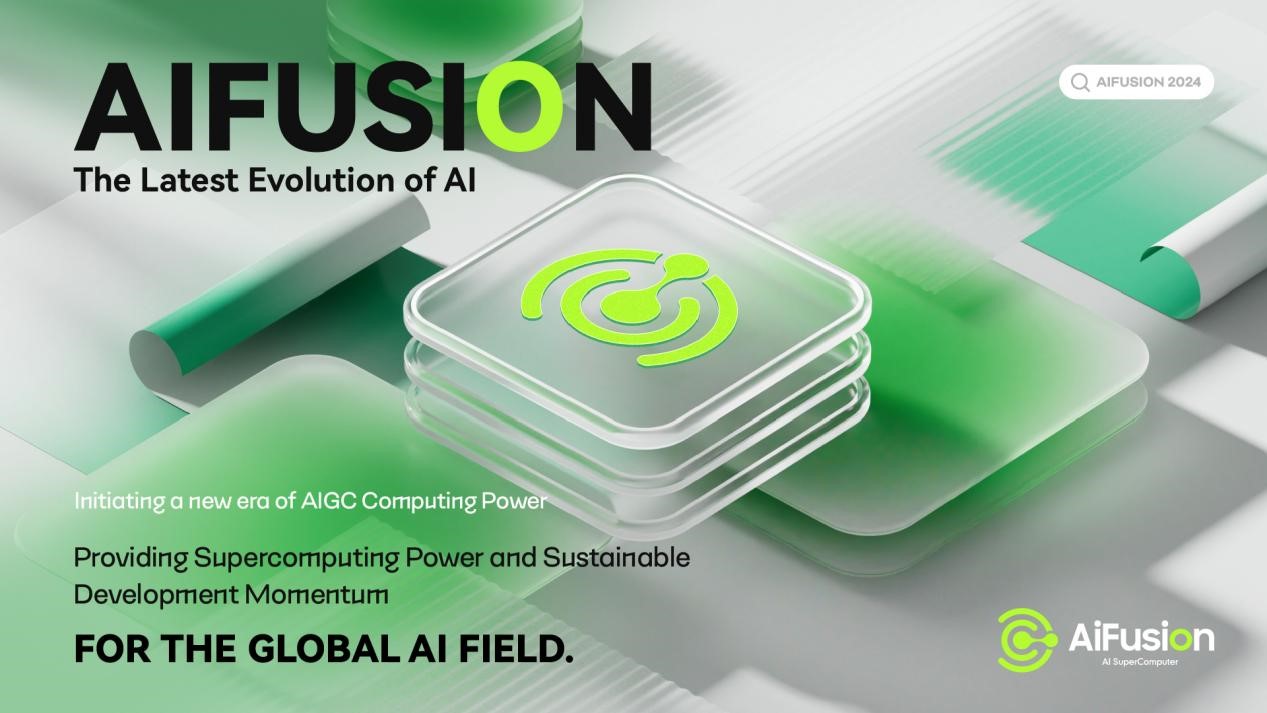
AIFusion: igniting a new supercomputing spark in the field of AI
Driven by the rapid development of technology and the trend of artificial intelligence, AIFusion was born, aiming to provide unprecedented cheap computing power and sustainable development super power for global AIGC entrepreneurs and developers in the AI field. This marks the official beginning of the supercomputing revolution in the AI era, injecting new vitality into the entire artificial intelligence industry.
AIFusion is not only a platform, but also a global experiment, committed to promoting the development of the artificial intelligence industry through its unique distributed computing power cluster network and computing power rental services under a trusted network. The platform provides global investors with convenient participation methods and AI income paths, and provides AIGC entrepreneurs and developers with an innovative and efficient computing power support system.
The AIFusion supercomputing protocol is at the heart of this revolutionary experiment. This agreement allows users to purchase AI supercomputing network mapping servers, follow the mining distribution mechanism of the agreement, and enjoy the computing power income dividends generated by the corresponding servers. This unique incentive mechanism not only allows users to fully enjoy advanced computing power services, but also becomes a powerful participant in the core computing power supply chain of the global AIGC industry.
AI Supercomputer, as a distributed supercomputing network platform launched by AIFusion, is jointly built by AIFusion Labs and Inflection AI, the founder of the foundation. The platform purchases computing power through centralized supercomputing equipment suppliers in 20 countries and regions around the world, providing stable and low-cost computing power rental, technology expansion and analytical data business support for AIGC industrial institutions and AI generation systems. This provides global users with the opportunity to participate in the core computing power supply chain of the AIGC industry, allowing them to obtain considerable returns on investment in the computing power blue ocean market.
The launch of the AIFusion supercomputing protocol means that global investors have a more open, inclusive, and shared opportunity to participate more deeply in the core of the artificial intelligence industry. This is not only a technological change, but also a revolution that connects the world with new sparks of AI supercomputing.
Under the leadership of AIFusion, we have witnessed the supercomputing revolution in the AI era and look forward to this innovative and dynamic platform bringing more surprises and breakthroughs to the field of artificial intelligence. AIFusion helps AI dreams soar and creates a new era of supercomputing!